How the ProcessMiner™ AutoPilot Platform Works
ProcessMiner™ is a decision automation platform that uses real-time artificial intelligence to help reduce energy consumption, raw materials, chemical usage and cost.
The Future of Manufacturing is Here
The first step in the optimization process is to access the historical data kept on the server and analyze it for data quality and consistency. This analysis will determine if the data collected is clean and consistently formatted for the purpose of optimizing the manufacturing process. Our industry-leading autonomous control solution delivers process improvement recommendations and parameter control changes in real-time to the production line. Our platform ensures reductions in scrap, defects and errors commonly encountered in complex manufacturing processes.
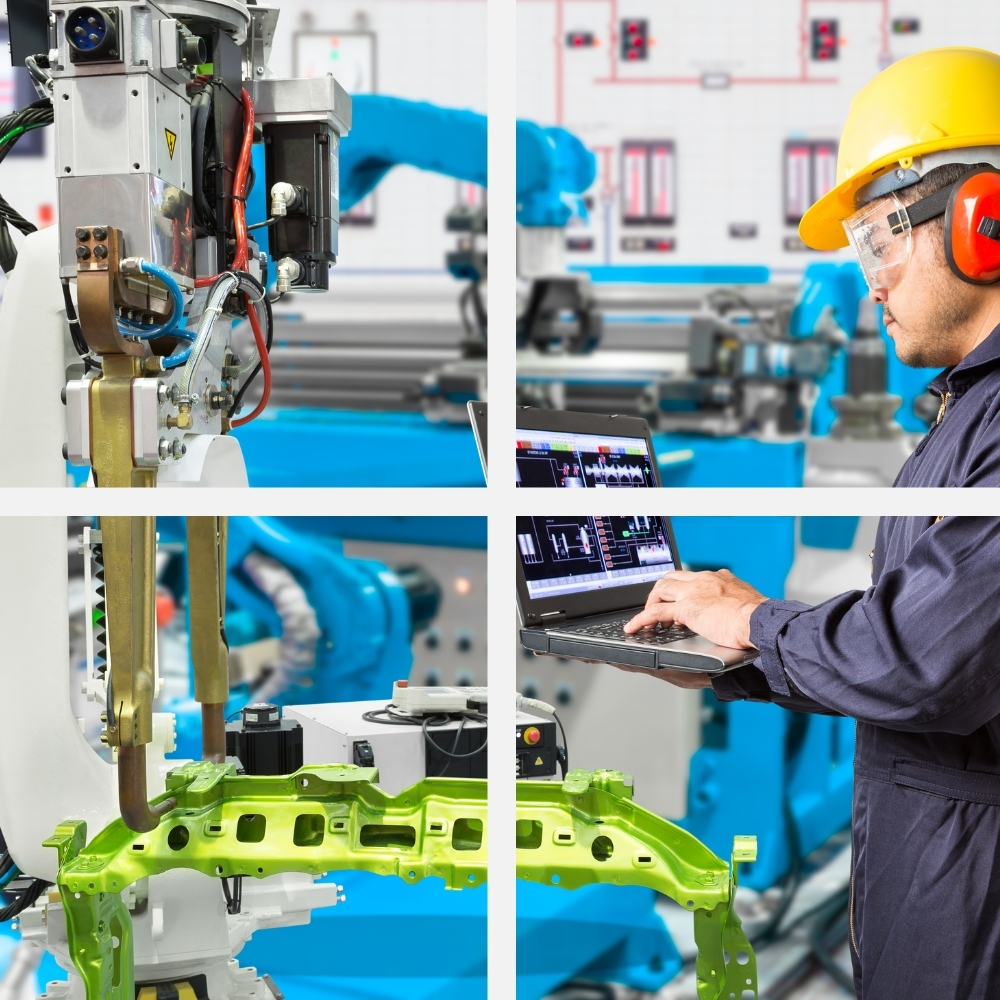
Continuous Quality Improvement is Our #1 Goal
ProcessMiner’s artificial intelligence platform is revolutionizing the manufacturing industry to deliver best-in-class quality, reduce waste, optimize sustainability and maximize bottom lines.
The ProcessMiner™ AutoPilot real-time predictive system comes in and solves problems before they happen, making recommendations and prescribing solutions. In doing this, it minimizes raw materials and reduces costs while maintaining both speed and product quality.
Autonomous Manufacturing Made Simple
The ProcessMiner™ AI platform is the industry leading autonomous control solution delivering automated process improvement recommendations and parameter control changes digitally and in real-time to machines and production lines.
Monitor
Monitoring historical data ensures you’re not just collecting data, but you’re collecting clean, relevant, trustworthy and actionable data to relay back to the server for quality and consistency.
Predict
Machine-learning system begins predicting performance measures and identifying improvement opportunities.
Recommend
Easy to read suggestions for minimizing variability through improvement, preventative and corrective actions.
Closed Loop
Through our predictive machine learning and artificial intelligence systems, real-time recommendations are automatically sent for autonomous manufacturing.
Optimize
Continual improvement optimizes process performance and variability. The longer it’s used, the more it improves.
Learn
Applies advanced data science, machine learning techniques to the manufacturing process to find insights and impact ROI.
Turnkey Artificial Intelligence Platform
ProcessMiner’s AutoPilot platform feature, in real-time, the quality of a product during the manufacturing process, makes corrective-action recommendations on that quality, and autonomously applies those recommendations using AutoPilot.
ProcessMiner’s turnkey, real-time dashboard and intelligent information, enables industries to thrive with reduced costs, reduced waste of raw materials, and maximized production outputs.
Monitor
Ensures you’re not just collecting data, but you’re collecting clean, relevant and actionable data.
Before any analysis and modeling can happen, the data collected in a manufacturing plant needs to be “clean.” The right sensors must be placed in the right locations, transmitting their data and relaying the information back to a server where a database stores the records. Some manufacturers may collect data from hundreds of sensors but they use only a portion of the information.
The first step in the optimization process is to access the historical data kept on the server and analyze it for data quality and consistency. The analysis will determine if the data collected is “clean” and “consistently formatted” for the purpose of optimizing the manufacturing process or whether any adjustments need to be made.
For example, the data assessment may uncover the need to replace a faulty sensor, or it may discover a gap in the data collection that needs to be filled. The industry and manufacturing expertise at ProcessMiner™ helps to guide the analysis of the historical data and determine what’s needed to make sure the information is useful and trust-worthy.
Predict
Predicts performance measures and identifies improvement opportunities.
With the modeling in place, the process moves to the prediction phase where the machine-learning system begins using data from real-life manufacturing to forecast what will happen during the actual production run.
Monitoring occurs with artificial intelligence running in the background and can predict the outcomes in the future as opposed to other systems that simply react to what has already occurred during the manufacturing process.
The difference allows ProcessMiner™ to provide plant owners and operators with real-time actionable recommendations to improve operational efficiency and product quality.
Recommend
Easy to read suggestions for minimizing variability though improvement, preventative and corrective actions.
For recommendations, operators have access to web-based interfaces that display data generated by the ProcessMiner™ platform.
On the screen, the operators can view several key performance metrics along with suggestions for adjusting certain inputs to improve results. The recommendations are provided based on their potential impact and are regularly updated during the production run.
If the operator decides to implement the recommendation, the alert disappears once the system recognizes the change and observes improvement in the manufacturing process.
With each observation, adjustment and recommendation, the ProcessMiner™ machine learning program gains more knowledge of the manufacturing process and uses this data to make future predictions and forecasts. In fact, it uses this knowledge to optimize the process over time.
Closed Loop
ProcessMiner’s AI platform is the industry leading autonomous control solution delivering automated process improvement recommendations and parameter control changes digitally and in real-time to machines and production lines.
This unique feature of our platform called AutoPilot delivers material reductions in scrap, defects and errors commonly encountered in complex manufacturing processes.
The ProcessMiner™ AutoPilot real-time predictive system comes in and solves problems before they happen, making recommendations and prescribing solutions. In doing this, it minimizes raw materials and reduces costs while maintaining both speed and product quality.
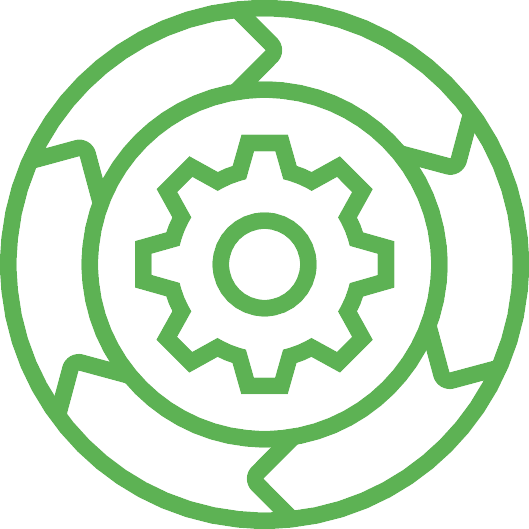
Optimize
Continual improvement that optimizes process performance and variability. The longer it’s used, the more ProcessMiner™ improves.
The longer it’s used, the more ProcessMiner™ improves. Its machine learning and artificial intelligence adjust for the constantly changing data the sensors relay from the manufacturing process because the data and the manufacturing conditions must be ongoing if the system is expected to provide predictions for the future.
Otherwise, the system just reacts to what’s already happened instead of preventing problems before they happen.
Each improvement raises the bar for ProcessMiner™, and thanks to the unique manufacturing experience of the team, the platform is expected to continually evolve and improve so the finished state is a fully-integrated system that delivers ongoing solutions within a dynamic environment.
Learn
Applies advanced data science and machine learning techniques to your manufacturing process to find insights and impact ROI.
With good, clean data in hand, the next step is to learn from the data and create models for finding the relationship between manufacturing process variables and parameters, machine settings, and key measures using machine learning techniques.
The modeling permits analysis of the manufacturing process without altering the real-life operations. It also allows data scientists to test theories of how the manufacturing process might change if certain elements such as temperature and pressure are adjusted. It can get complicated very quickly, especially when multi-response modeling is applied to the predictive analysis of manufacturing systems.
Often, the modeling will focus on a specific issue with a potential for a sizable return on investment. Sometimes, the business wants to use fewer raw materials in the manufacturing process, thereby reducing costs. Or, it may want to improve the quality scores for its products, which can lead to an increase in volume to be sold.
What separates the modeling at ProcessMiner™ from the competition is the integration of data-driven and domain-expertise solutions. The platform leverages deep industry knowledge and subject matter expertise obtained from decades of manufacturing process experience and adds a healthy dose of human intelligence to the artificial kind obtained from data. In practice, this leads to better insights and more focused optimizations that only people with specialized industry knowledge can offer.
Contact Our Data Science Team
Fill out the form below and we’ll get back to you, or contact us by phone to speak to a miner today.
Have a project in mind?
For more information, download our brochure. We’ll reach out to you!
U.S. Headquarters
75 Fifth Street NW
Suite 2505
Atlanta, GA 30308
+1 (678) 463-5799
info@processminer.com
International
INDIA
FINLAND
Oy ProcessMiner Ab
Otakaari 5
02150 Espoo
+358 400 450 748
jvanska@processminer.com